User contribution
Semi-automated prediction of behavioral states in wild understudied King vultures (Sarcoramphus papa)
18. December 2023
Authors
Chris Beirne | chrisbeirne@osaconservation.org | https://www.tropicalmovementlab.com
Tobias Petri | thp@firetail.de | https://www.firetail.de
Abstract
In this article, we examine the current gap between research-oriented and conservation-based approaches in the application of acceleration data for behavioral analysis.
We use high-quality, state-of-the-art e-obs data from King Vultures (Sarcoramphus papa) in Costa Rica (collected by Osa Conservation: https://osaconservation.org/) to show how to employ a semi-automated machine learning approach (FireSOM) embedded in the Firetail software to interactively and rapidly derive behavioral states from acceleration data. Our results highlight the benefits of inspecting predictions in the context of all available sensor data.
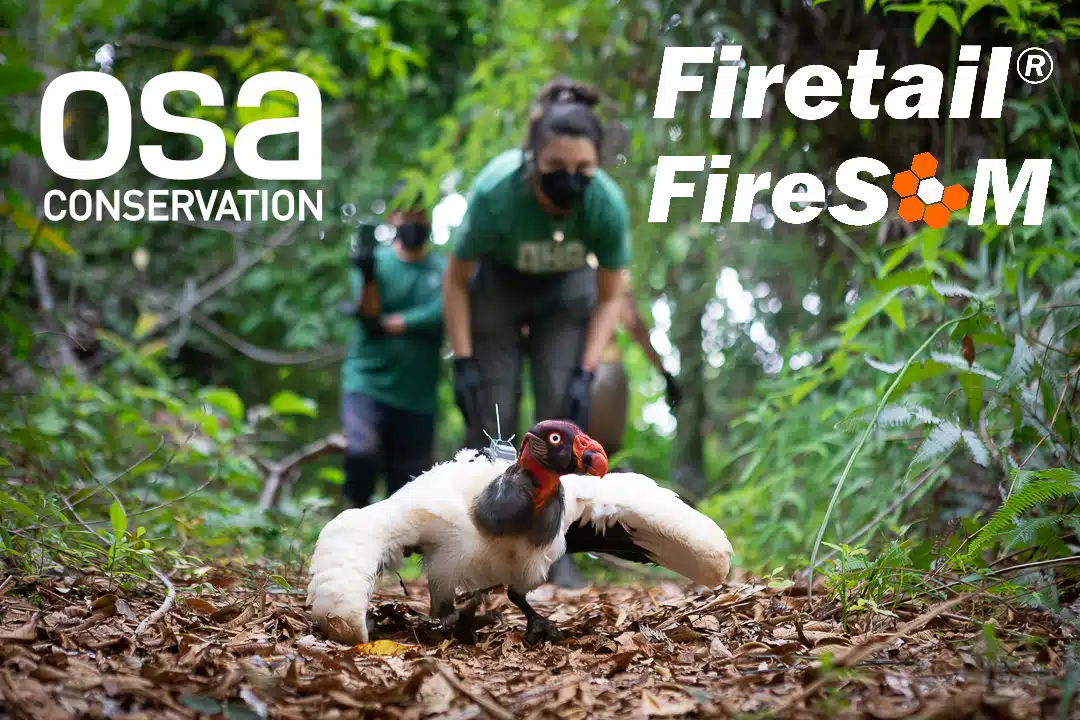
Acceleration data
While typical sensor events are in-sync with location fixes, other sensors allow for data independent of location. A well-known example is acceleration data, which measures the level of acceleration induced on a tag in three axes, X,Y and Z, independently. The data is commonly recorded as consecutive snapshots over multiple seconds, also known as `bursts`. Acceleration data might indeed help to improve a wide range of problems ranging from modeling of flight phases to deducing behavior and habitat selection crucial for wildlife ecologists and conservation practitioners. Few projects routinely exploit the full potential of these acceleration sensors, and there seems to be a gap among academic applications and applied modeling in conservation projects.
Complexity as a reason for obstruction
It is intuitive to interpret geolocation data for direct, model-free, analyses. Whereas, the principal reasons for the lack of widespread utilization of accelerometer data in conservation are: (1) the more complex, less intuitive nature of the data, and; (2) the higher data density making data management and manipulation intimidating. This makes acceleration data tricker to use and more difficult to communicate. One way to overcome these limitations and reduce data complexity is to use artificial intelligence (‘AI’) approaches to recognize recurring patterns in the data to cluster acceleration fragments. This allows tag owners to work at the level of higher-order acceleration categories, instead of raw data representations. This renders the accelerometer data more accessible, hopefully resulting in increased utilization for research and conservation contexts.

(Figure 1) The Firetail interface integrates interactive location visuals, acceleration measurements, sensor data and event sensor information via available timestamps. Behavioral annotation is done using the FireSOM module that detects abstract categories of acceleration patterns. These are shown as colorbars (top left) in sync with the acceleration data to inspect their context and finally assign behavior.
Reducing the complexity
Processing the amount of data that modern tags record is challenging, potentially excluding biologists and conservationists without an appropriate background.
The Firetail analysis platform (www.firetail.de, Figure 1) has been devised to make acceleration data more accessible and easily interpretable. It works interactively, combining location data, acceleration data and sensor events and relies on sophisticated access structures to handle huge datasets interactively without the requirement for coding.
Facilitating annotation of acceleration data
Assigning behaviors to bursts is complex in non-model organisms being tracked for the first time, as few reference libraries and databases of unified and standardized data sets exist. To facilitate the annotation process, Firetail’s FireSOM module was developed. Its core component is a self-organizing map (SOM, also Kohonen map[1]) that will assign each burst to a category. Visually, colored categories are organized in named layers and are shown above the acceleration data. They can be examined, renamed, deleted and merged interactively. Models can be saved and used to predict behavior in other individuals.
[1] Kohonen, Teuvo. “Essentials of the self-organizing map.” Neural networks 37 (2013): 52-65.
King vulture data
Here we apply the FireSOM workflow to a population of King vultures on the Osa Peninsula, Costa Rica (Figure 2). The birds were captured and tagged as part of Osa Conservation’s (https://osaconservation.org/) movement ecology program. Between February and September 2022, 18 individuals were tagged with 42g e-obs devices using wing-loop harnesses. The tags were programmed to record location and tri-axial acceleration data (20Hz) every ten minutes when birds were active, and every thirty minutes when inactive between the hours of 5 am and 7 pm. In addition to this, all birds were subject to a 10 day period of high resolution (location and acceleration data each minute) between the 14th and 28th of February (start dates varied by individual). All devices were still functioning as of December 2023. All data were accessed through Movebank (www.movebank.org, ‘OC Vultures’).
(Figure 2) King Vulture project – thermal uplift for individual King Kong – 1Hz e-obs data; track region selected and exported as KML using Firetail.
(Figure 3) Predicted categories for a takeoff event of individual Messi – acceleration burst boundaries are indicated by light gray vertical bars. The category ‘above4ms’ on the ‘speed_threshold’ layer is assigned using a threshold of 4m/s on the sensor data. In the ‘flight/flapping’ layer two flapping intensities are assigned based on the triaxial acceleration patterns (x|y|z = red|green|blue) using FireSOM. The layer ‘flight/soaring’ contains two similar categories of soaring flight.
Semi-automated Annotation Workflow
We selected an individual (‘Messi’) to train a model (Figure 3) which was then applied to the other 17 individuals (Figure 4). E-obs` tags provide reliable speed-over-ground measurements for each position. Therefore we could apply a threshold of 4 m/s to train two distinct FireSOM models for flying and non-flying states.
In the FireSOM workflow representative instances of each predicted category are inspected and all associated bursts are assigned to a behavioral state. The burst assignments can be evaluated in the context of all available data, including location where the acceleration burst was collected, satellite imagery to give context to the bird’s location, and the pattern of locations preceding and succeeding it.
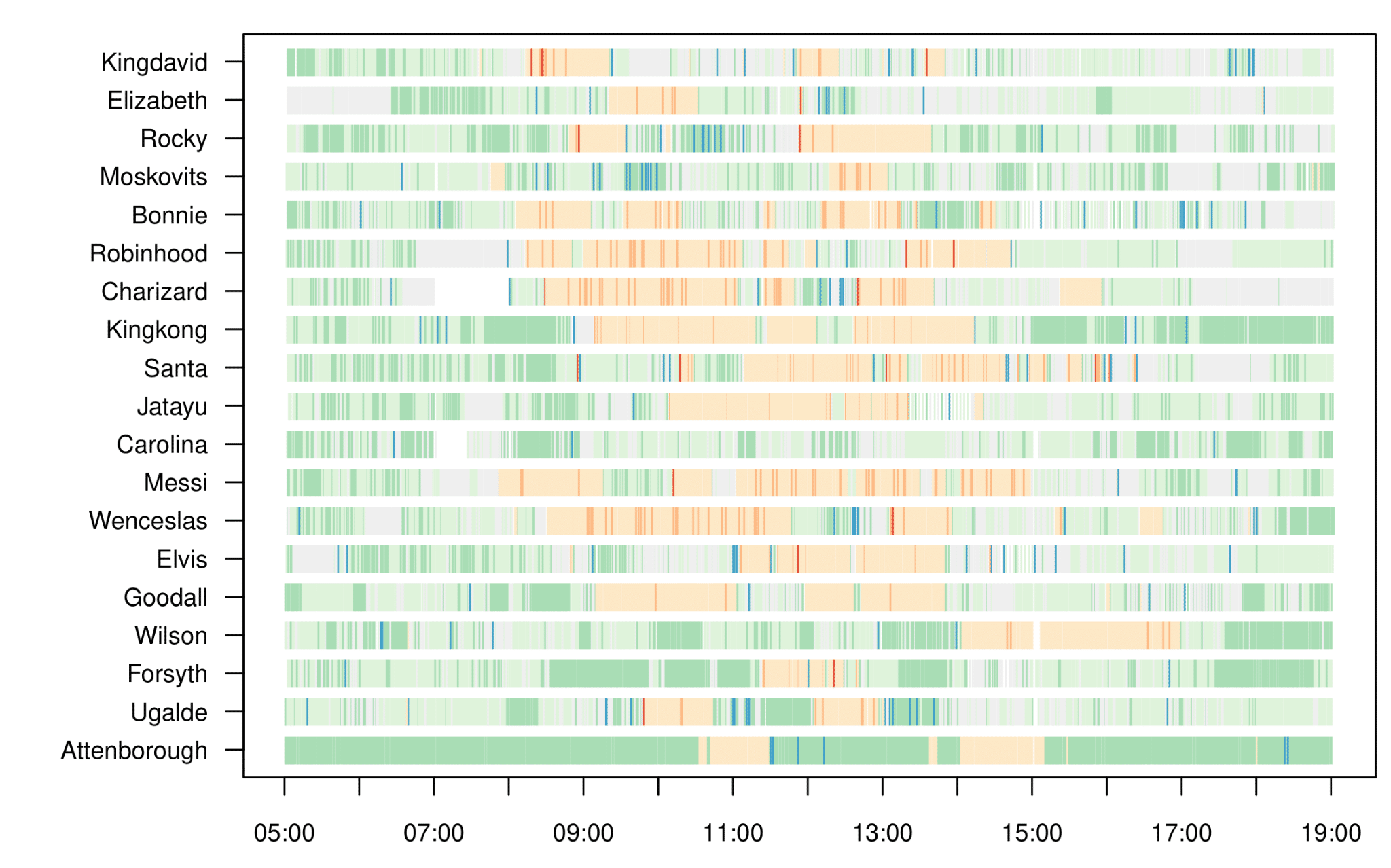
Figure 4, project-wide activity – results for the model transfer of behavioral categories for the king vulture datasets for a single day (19th of May 2023). light/dark orange: soaring/flapping, light/dark green: roosting/movement. It can be observed that King vultures typically are more active around midday (flying/hopping), typically have 1 to 2 flight ‘bouts’ per day, and spend very
Towards large-scale gold standards
Assigning behavioral categories to tracking data of wildlife which are rare or living in hard-to-access terrain is extremely difficult. Here, we highlighted how high-resolution acceleration sensor data (e-obs) can be embedded in a semi-automated acceleration segmentation workflow (FireSOM, www.firetail.de) which can help to rapidly assign behavioral categories in a poorly understood species, the King vulture. The pattern-driven approach can help to shed light on otherwise hidden behaviors. Our workflow enables the step-wise overlay of gold-standards and intrinsic evaluation to incrementally improve and refine the initial models.
Firetail can assist this process by an interactive toolbox of algorithms and modules to integrate these data. While the proposed models are far from perfect or complete, our proposed method can rapidly enable experiment-wide acceleration data annotations with no requirement for large-scale labeling of data. This work promotes the integration and rigorous evaluation of predictions by available gold standards (e.g. animal borne video devices) and can contribute to the development of species-specific, pattern-based behavioral databases.